Table of Contents
Introduction
Machine learning (ML) continues to revolutionize industries and reshape the way we interact with technology. As we move into 2024, several emerging trends are set to drive the evolution of machine learning. Understanding these trends is crucial for businesses and professionals aiming to stay ahead in this rapidly changing field. Here, we delve into the most significant machine learning trends to watch in 2024.
Democratization of Machine Learning
In 2024, the democratization of machine learning will gain momentum. As tools and platforms become more user-friendly, individuals without extensive technical backgrounds will have the ability to create and deploy machine learning models. This shift will be fueled by:
Automated Machine Learning (AutoML): AutoML platforms, such as Google’s AutoML and Microsoft’s Azure Machine Learning, enable users to automate the process of model selection, hyperparameter tuning, and feature engineering. These platforms make it easier for non-experts to develop high-quality models.
No-Code and Low-Code Platforms: Tools like DataRobot and H2O.ai offer no-code and low-code solutions that simplify the development process, allowing business analysts and domain experts to build machine learning models without writing code.
Enhanced Explain-ability and Transparency
With the growing use of machine learning in critical applications, the need for explain-ability and transparency has become paramount. In 2024, we expect to see advancements in:
Explainable AI (XAI): Techniques such as SHAP (SHapley Additive exPlanations) and LIME (Local Interpretable Model-agnostic Explanations) will continue to evolve, providing clearer insights into model predictions and decision-making processes.
Regulatory Compliance: New regulations and guidelines will push for greater transparency in AI systems. This will drive organizations to adopt explain-ability tools to ensure compliance and build trust with users.
Federated Learning and Privacy-Preserving Techniques
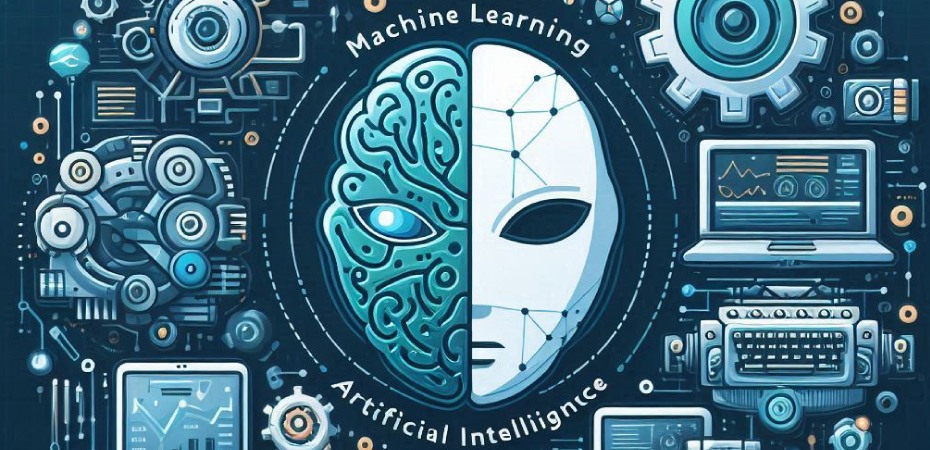
As concerns about data privacy and security grow, federated learning and other privacy-preserving techniques will become more prominent. These approaches enable machine learning models to be trained across multiple decentralized devices or servers without sharing raw data. Key developments include:
Federated Learning: Initiatives by Google, Apple, and other tech giants are advancing federated learning methods that allow models to learn from data distributed across multiple locations while keeping the data localized and secure.
Differential Privacy: Techniques that add noise to data to preserve individual privacy while allowing aggregate analysis will see increased adoption, particularly in sectors like healthcare and finance.
Integration with IoT and Edge Computing
The integration of machine learning with Internet of Things (IoT) and edge computing will transform various industries by enabling real-time data processing and decision-making at the edge. This trend will be characterized by:
Edge AI: Deploying ML models on edge devices such as sensors, cameras, and smart appliances to perform local data analysis. This reduces latency and bandwidth usage, making applications faster and more efficient.
IoT Data Analytics: Leveraging ML algorithms to analyze data from IoT devices in real-time, enabling predictive maintenance, anomaly detection, and enhanced operational efficiencies in sectors like manufacturing, logistics, and smart cities.
Quantum Machine Learning
Quantum computing is poised to revolutionize machine learning by solving complex problems faster than classical computers. In 2024, we anticipate significant strides in:
Quantum Algorithms for ML: Development of quantum algorithms that can accelerate tasks such as optimization, sampling, and matrix operations, which are fundamental to machine learning.
Hybrid Quantum-Classical Systems: Combining quantum and classical computing resources to create hybrid systems that can tackle large-scale ML problems more efficiently than traditional methods alone.
Ethical AI and Bias Mitigation
As machine learning becomes more pervasive, addressing ethical concerns and mitigating bias in AI systems will be crucial. In 2024, we will see:
Bias Detection and Mitigation: Enhanced techniques for detecting and mitigating bias in ML models, ensuring fair and equitable outcomes. Tools like Fairness Indicators and AI Fairness 360 will play a significant role in this area.
Ethical Frameworks: Organizations and governments will develop and adopt ethical frameworks and guidelines to govern the use of AI. These frameworks will emphasize accountability, transparency, and fairness in AI development and deployment.
Advances in Natural Language Processing (NLP)
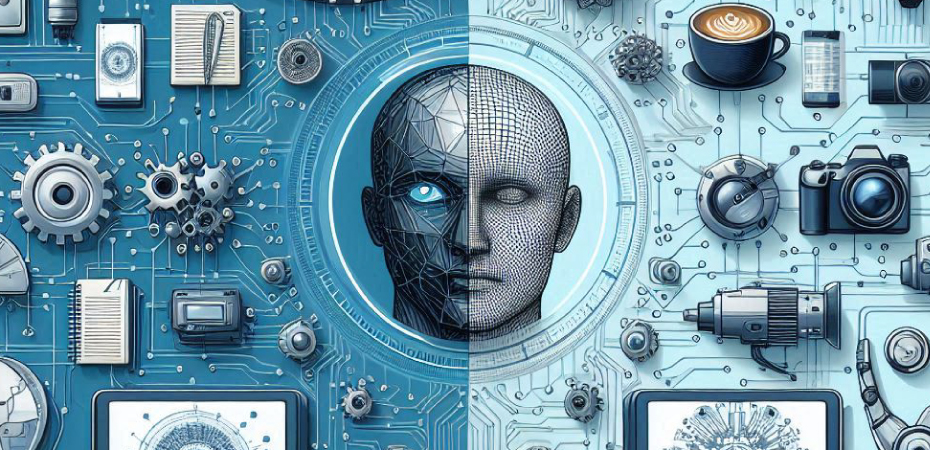
Natural Language Processing (NLP) will continue to advance, making interactions between humans and machines more seamless and intuitive. Key trends in NLP for 2024 include:
Transformers and Large Language Models: Models like GPT-4 and BERT will be further refined, enabling more accurate and context-aware language understanding and generation.
Multilingual and Low-Resource Language Processing: Improved techniques for processing languages with limited training data, expanding the reach and applicability of NLP technologies globally.
Reinforcement Learning and Autonomous Systems
Reinforcement learning (RL) will drive innovation in autonomous systems, robotics, and gaming. In 2024, expect to see:
Advancements in RL Algorithms: Development of more efficient and scalable RL algorithms that can learn complex behaviors in dynamic environments.
Applications in Autonomous Systems: Increased deployment of RL in autonomous vehicles, drones, and industrial robots, enhancing their ability to navigate and make decisions in real-time.
Personalized and Adaptive Learning Systems
Personalized learning systems will become more sophisticated, leveraging machine learning to tailor educational content and experiences to individual needs. In 2024, trends will include:
Adaptive Learning Platforms: Tools that use ML to adjust the difficulty and type of content based on a learner’s progress, providing a customized educational experience.
AI Tutors: Intelligent tutoring systems that offer real-time feedback and support, enhancing the learning process and outcomes for students.
Sustainable and Green AI
As the environmental impact of AI becomes a growing concern, the focus on sustainable and green AI will increase. In 2024, we anticipate:
Energy-Efficient Algorithms: Development of algorithms that require less computational power and energy, reducing the carbon footprint of AI operations.
Sustainable AI Practices: Adoption of practices that promote sustainability in AI research and deployment, such as optimizing data center energy use and leveraging renewable energy sources.
Conclusion
The future of machine learning in 2024 promises to be exciting and transformative. By staying abreast of these trends, businesses and professionals can harness the power of machine learning to drive innovation and achieve competitive advantage.
21 thoughts on “The Future of Machine Learning | Trends to Watch in 2024”
Hello, Jack speaking. I've bookmarked your site and make it a habit to check in daily. The information is top-notch, and I appreciate your efforts.
The posts inspire me regularly. The depth you bring to The topics is truly exceptional.
This is the most thorough piece I’ve read on the topic. The dedication to research is admirable.
Presented a hard to understand topic engagingly, like a magician pulling a rabbit out of a hat.
Unique viewpoints, because who needs echo chambers?
You tackled a hard to understand issue with elegance and insight. I feel much more informed after reading The post.
The creativity and intelligence shine through this post. Amazing job!
You have a gift for explaining things in an understandable way, much like a smooth talker who knows just what to say.
Pingback: 5 High-Demand Soft Skills That Help You Gain a Remote Job - Study Guide Courses
Reading The article was a joy. The enthusiasm for the topic is really motivating.
Truly inspirational work, or so I tell myself as I avoid my own projects.
The balance and fairness in The writing make The posts a must-read for me. Great job!
Touched on personal resonances, or as I like to call it, psychic abilities.
Thanks for the hard work. I could almost see the sweat on the keyboard. Much appreciated!
I’m amazed by The knowledge, almost as much as I’m drawn to the way you present it. Share more, please?
The expertise and hard work shine through, making me admire you more with each word.
NY weekly I truly appreciate your technique of writing a blog. I added it to my bookmark site list and will
The depth you bring to The topics is like diving into a deep pool, refreshing and invigorating.
New cryptocurrency releases
Engaging with The work is as thrilling as a spontaneous road trip. Where to next?
Your article helped me a lot, is there any more related content? Thanks!